Deep learning is a complicated process that’s fairly simple to explain. A subset of machine learning, which is itself a subset of artificial intelligence, DL is one way of implementing machine learning (automated data analysis) via what are called artificial neural networks — algorithms that effectively mimic the human brain’s structure and function. And while it remains a work in progress, there is unfathomable potential. In this article, we’ll briefly explain how deep learning works and introduce the best companies today.
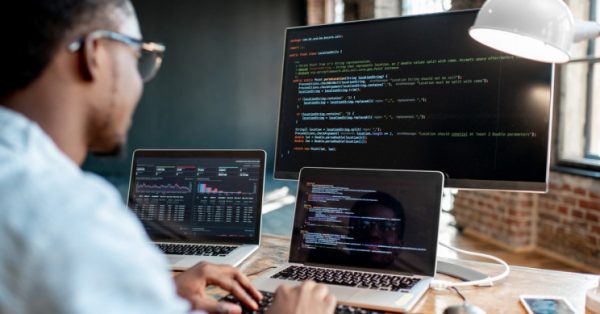
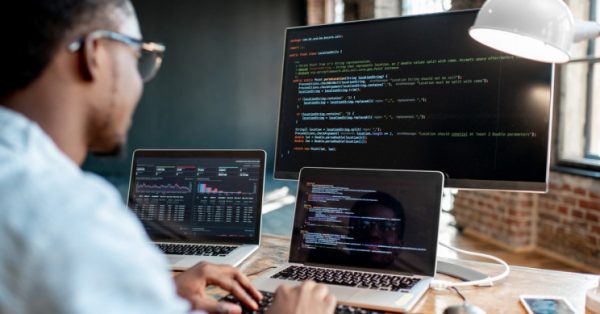
What Is Deep Learning?
Deep learning is a subcategory of machine learning methods powered by artificial intelligence technologies. These systems first develop a deep domain insight and then provide this information to the end-users in a timely, natural, and usable way. Since there is a rapid increase in data generation across industry verticals such as banking, financial services, and insurance (BFSI), healthcare, retail, and public services, the adoption of deep learning is also growing fast.
Currently, almost all organizations are using machine learning algorithms to make their business functions effective and efficient. As the same way, Artificial intelligence and machine learning companies try to deliver a robust service to their customers by using machine learning as their prominent business principle. Generally, machine learning and artificial intelligence companies used this technique for integrating multiple sources of data or a vast amount of data, data security, real-world applications, prediction, cloud operations, etc.
Deep Learning Examples
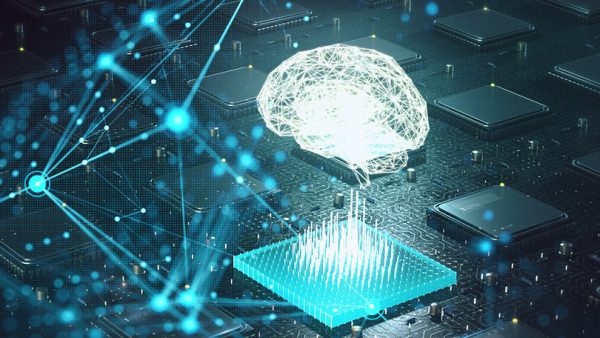
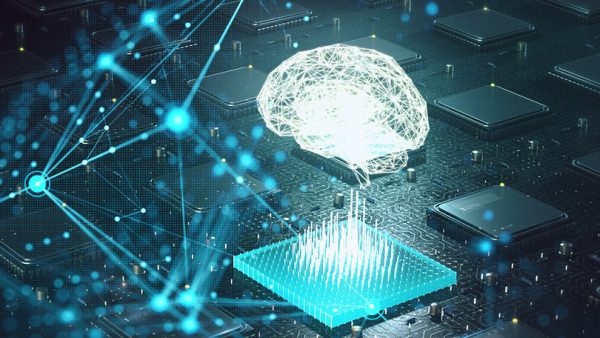
The major deep learning examples are the implements of AI-enabled systems to make human tasks more efficient and accurate. For instance, in the financial sector, deep learning systems help bank employees extend their work capabilities and allow financial institutions to concentrate more on customer interaction rather than the traditional transaction-based approach. Furthermore, based on the client’s history and background, the deep learning systems can offer contextual and evidence-based reasoning by engaging in dialogue with humans. Deep learning systems also enable customized and self-service options for customers. Moreover, it assists employees in offering tailored recommendations for customer specifications.
Other latest deep learning examples can be seen in various areas, such as virtual assistants (Alexa or Siri), language translations, Chatbots, facial recognition, personalized push notification, remote monitoring & controlling and more.
Deep Learning Market Trends
The US will continue to hold the maximum market share for the next few years as it the home of many world’s top deep learning companies. According to the recent market research from Technavio, the US deep learning market size is forecast to grow rapidly at a CAGR of more than 57% by 2021.
The demand for AI & Machine Learning is exploding—from the startups advancing it to the job-seekers chasing it to the growing tech community watching its every move.
Deep Learning Jobs
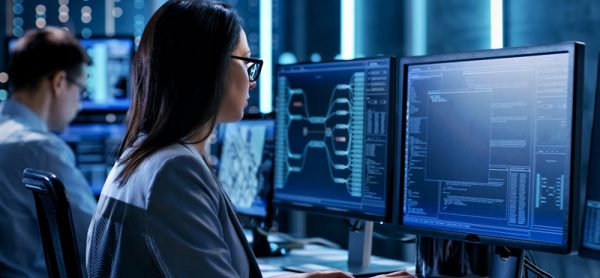
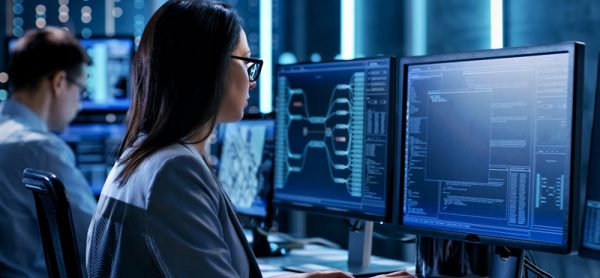
Artificial intelligence is one of the most exciting and attractive fields to get into. The global machine learning (ML) market is estimated to grow from $1.4 billion in 2017 to $8.8 billion by 2022. AI is projected to create 2.3 million related jobs by 2020, according to Gartner. The average salary of a machine learning engineer is between $125,000 and $175,000. At the top ten highest paying companies for AI talent, the average salary easily surpasses $200,000. Clearly, there are a lot of reasons to join this booming industry.
There are traditionally two fundamental splits here when you’re working with datasets and artificial intelligence/machine learning:
Data scientists
Basically, data scientists help communicate findings from data models to business decision-makers. Moreover, they help tune and tailor models that help businesses ask the right questions of their data.
Machine Learning Engineers
Build the data plumbing that allows for data scientists to process huge amounts of data. In practice, they’re responsible for feeding the models defined by data scientists. Also, they’re often responsible for taking theoretical data science models and helping scale them out to production-level models that can handle the day-to-day of companies that generate terabytes of data.
5 Deep Learning Companies To Keep An Eye On
1. NVIDIA
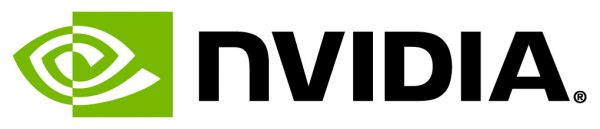
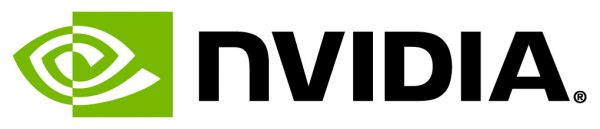
NVIDIA AI Platform for Developers’ GPU-accelerated deep learning frameworks offer flexibility to design and train custom deep neural networks and provide interfaces to commonly-used programming languages such as Python and C/C++, making NVIDIA one of the world’s leading deep learning developers.
2. Sensory
Sensory is a Silicon Valley based AI and deep learning software company, focusing on providing products and services to improve the user experience through embedded machine learning technologies such as voice, vision, and natural language processing. Moreover, Sensory’s TrulySecure uses a deep learning approach in our face and voice recognition algorithms.
3. Qualcomm
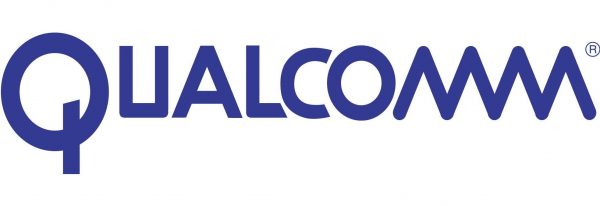
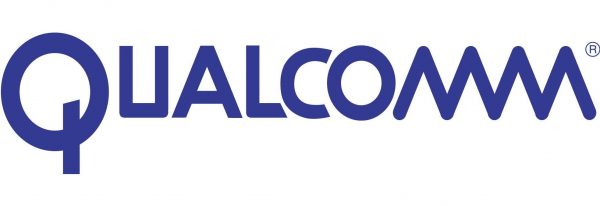
Qualcomm Incorporated is one of the world’s leading telecom companies, headquartered in San Diego. They offer AI and deep learning solutions for various end-devices including smartphones, machines and vehicles. It also uses deep learning techniques for power-efficient implementations across hardware, algorithms, and software.
4. Amazon
Amazon SageMaker provides developers and data scientists with the ability to build, train, and deploy machine learning models quickly. This is a service that covers the entire machine learning workflow. These include label and prepare data, choose an algorithm and train the model. Finally, SageMaker is able to make predictions, and take action.
5. Microsoft
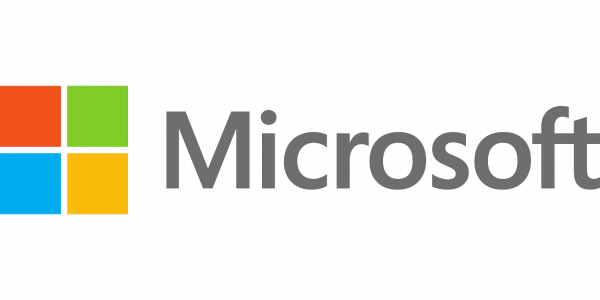
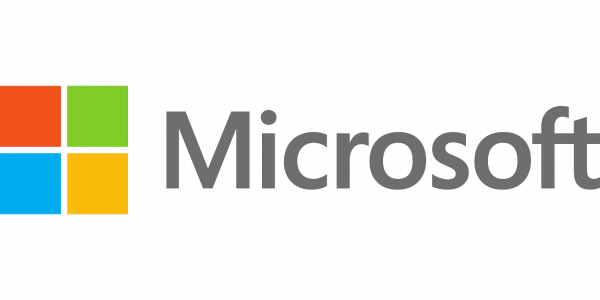
The Microsoft Cognitive Toolkit (CNTK) is an open-source toolkit for commercial-grade distributed deep learning. CNTK allows the user to easily realize and combine popular model types. These include feed-forward DNNs, convolutional neural networks (CNNs) and recurrent neural networks (RNNs/LSTMs). CNTK also implements stochastic gradient descent (SGD, error backpropagation) learning with automatic differentiation and parallelization across multiple GPUs and servers.