The financial industry handles a vast amount of data every minute. Thus, machine learning in finance has proved to be extremely beneficial in its services and operations. Aside from enhancing financial analyses and improving their security, banks and other financial institutions are adapting and developing this technology further.
To understand what it is and its application in finance, we’ll take a closer look at machine learning.
What Is Machine Learning?
Machine learning is a subset of artificial intelligence (AI). It uses algorithms and statistical models to perform a task without being explicitly programmed to do so. This means that computer systems can make predictions and decisions based on the data fed in it. It learns from experience and relies on patterns to make inferences.
Machine learning in finance works extremely well. This is because the more data you feed, the more accurate the results will be. Additionally, financial institutions handle enormous amounts of data every minute: bank transactions, customer data, credit bills, and a whole lot more. Therefore, machine learning is very valuable in finance.
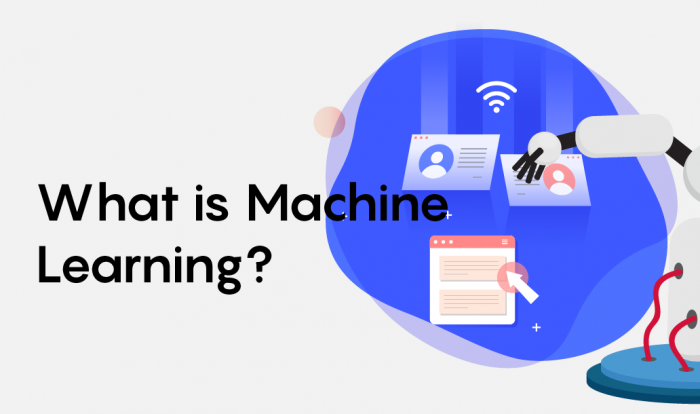
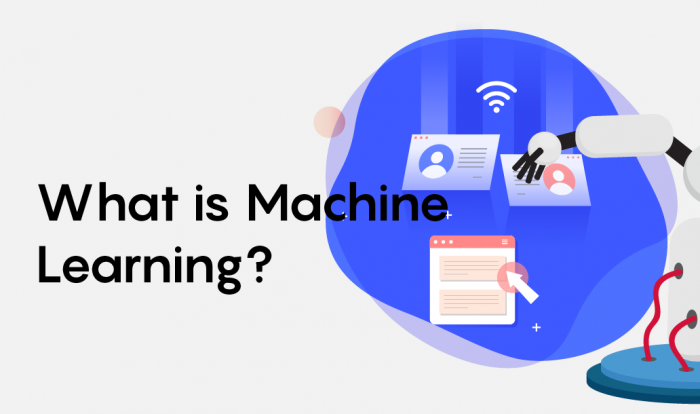
Machine Learning Applications In Finance Today
It is difficult to imagine the future of finance without machine learning. At present, financial institutions are currently leveraging AI technology (which includes machine learning) in their operations. Below are some of the machine learning applications in finance today.
Portfolio Management And Optimization
To efficiently manage their clients’ portfolios, financial institutions are using Robo-advisers. Contrary to its name, it doesn’t use robots to perform its services. Rather, it is an online application that provides guidance and manages portfolios for the clients. The use of Robo-advisers and machine learning investment management have become popular today because they have cheaper fees compared to human advisors.


Fraud Prevention And Detection
Banking and financial institutions sit at the top among the industries that fraud affected the most. The cost of recovery in every fraudulent activity is greater than the money lost to fraud. Thus, machine learning in finance is a big help in preventing and detecting fraud. Sophisticated algorithms can spot patterns and block fraudulent activities accurately.
Algorithmic Trading
Algorithmic trading uses algorithms to place trades without requiring human intervention. The trader can specify a set of parameters such as price, quantity, and timing for the algorithm. Then, the computer can place a trade autonomously based on the algorithm. It simplifies the decision-making process and saves time through automation. Currently, machine learning algorithms for trading are being developed to calibrate better trading decisions.


Predictive Analytics In Marketing
Marketing is an important aspect of finance. It also involves a massive amount of data where deep learning in finance comes in. A machine learning software can predict the effectiveness of a marketing strategy by analyzing previous ad campaigns, web activity, and mobile app usage of customers. This is extremely helpful in creating better ad campaigns and marketing strategies in the future.
Loan And Insurance Underwriting
Insurance companies also benefit from machine learning in finance underwriting. Using algorithms, companies can predict whether an applicant qualifies for insurance. They can also calculate risks, determine premiums, and match data records through machine learning. It also works with loan underwriting.
Risk Management
As much as possible, financial institutions avoid risks and crisis that could affect them. This is why financial risk management is using machine learning. Aside from predicting creditworthiness, machine learning in finance can also analyze current market forecasts, high-impact events, and critical information. This helps financial institutions manage upcoming risks to predict the financial crisis.
Digital Assistants
Virtual assistants are popular today, and they do get the job done. But in the financial world, digital assistants are making waves as well. Some of the most well-known ones are Amazon Alexa, Apple’s Siri, and Microsoft’s Cortana. Machine learning for financial engineering allows digital assistants to analyze their user’s needs and behavior so they can operate efficiently. They are also easily accessible at any minute.
Customer Service
Excellent customer service is one of the keys to a successful financial institution. When a bank has terrible customer service, clients are likely to botch them and find a better company. There is also an application of machine learning in finance in customer service. Ordinary chatbots have a limited amount of information and cannot solve a customer’s problem efficiently. However, machine learning-enabled chatbots can learn and solve a customer’s query based on their behavior.
Document Analysis
Deep learning in finance is revolutionizing the way financial institutions are doing legal work. What used to require thousands of hours of manual work can now be completed in seconds, thanks to machine learning. J.P. Morgan Chase Bank has invested in the development of Contract Intelligence (COiN) for document analysis. It can interpret documents in just seconds, taking off a huge amount of workload for its attorneys.
Trade Fails
Trade settlement is the process of transferring payment and securities to following a stock trade. This process is entirely electronic. However, trade fails can still occur so there’s a need for manual resolving. To avoid trade fails, machine learning in finance is utilized. It can pinpoint the reason why the trade has failed, provide a solution, and predict future trade fails. Instead of waiting for several minutes for a human to resolve a trade fail, machine learning can fix it in a matter of seconds.
What Is The Future Of Machine Learning In Finance?
While today’s machine learning applications are changing the way banking is done, developing and improving is still possible. Here are some of the future applications and innovations that machine learning can contribute to financing.
Enhanced security will be made possible. Instead of using usernames and passwords in online banking, stronger security features will be utilized. This includes facial recognition, biometrics, or voice recognition.
Customer service will possibly improve with machine learning as well. Chatbots can offer a more personalized service by pulling in their existing data. They can also provide recommendations and solutions based on patterns and behavior.
It is also possible that Robo-advisers will be developed and improved as well. They will be able to analyze a client’s goals, purchase trends, and savings trends better than before. As a result, more accurate recommendations can be provided.